Your cart is currently empty!
The Role of Recurrent Neural Networks in Autonomous Driving Technology
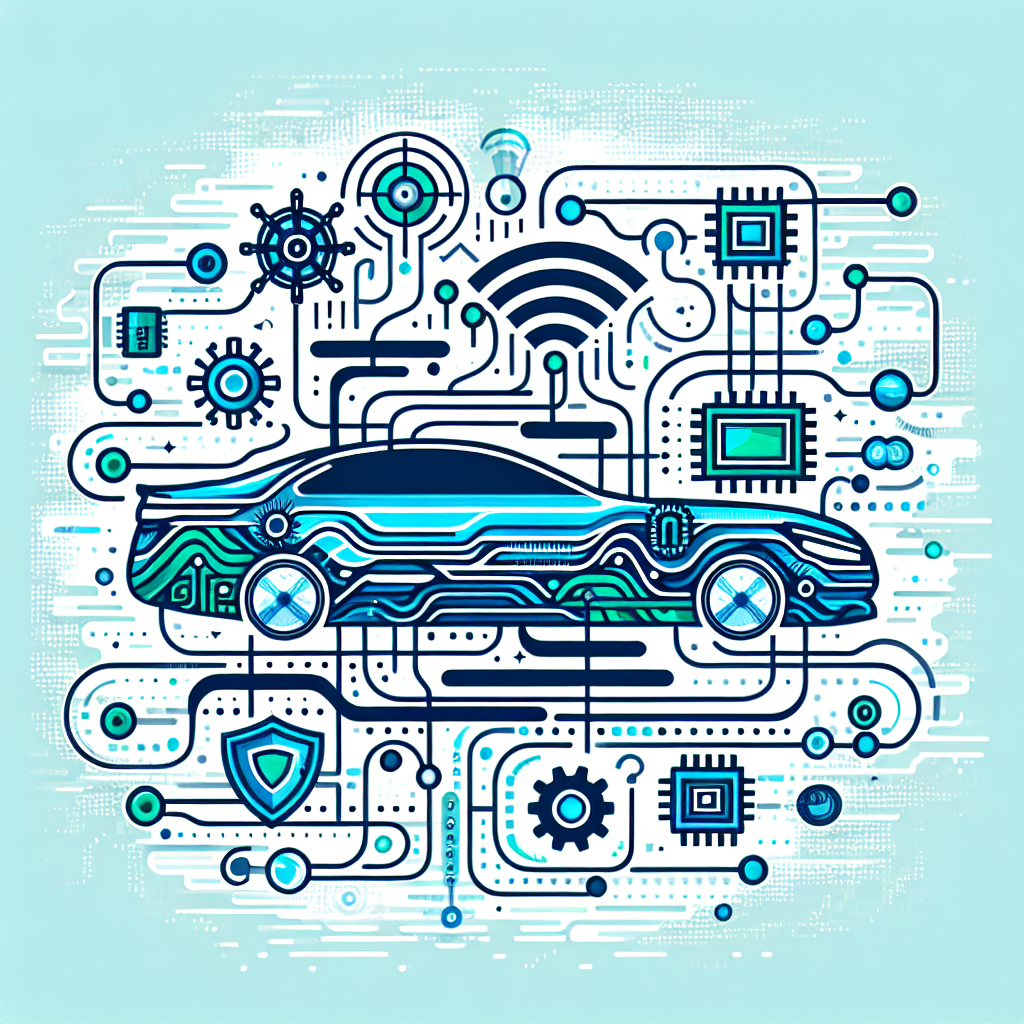
Autonomous driving technology has made significant advancements in recent years, with the development of sophisticated algorithms and machine learning techniques playing a crucial role in enabling vehicles to navigate roads safely and efficiently. One key technology that has revolutionized autonomous driving is recurrent neural networks (RNNs).
RNNs are a type of artificial neural network that is designed to handle sequential data and make predictions based on past information. This makes them well-suited for tasks that involve time series data, such as speech recognition, language translation, and, importantly, autonomous driving.
In the context of autonomous driving, RNNs are used to process inputs from sensors such as cameras, lidar, and radar, and make real-time decisions about steering, acceleration, and braking. By analyzing sequential data from these sensors, RNNs can predict the future trajectory of the vehicle and adjust its actions accordingly to navigate safely through traffic, avoid obstacles, and follow traffic rules.
One of the key advantages of RNNs in autonomous driving is their ability to handle complex and dynamic environments. Unlike traditional algorithms that rely on pre-defined rules and heuristics, RNNs can adapt to changing road conditions, unexpected obstacles, and unpredictable behaviors of other vehicles. This flexibility allows autonomous vehicles to respond quickly and accurately to new situations, making them safer and more reliable on the road.
Another important aspect of RNNs in autonomous driving is their ability to learn from experience. By training on large datasets of real-world driving scenarios, RNNs can improve their performance over time and become more adept at handling different driving conditions. This continuous learning process is essential for autonomous vehicles to achieve higher levels of autonomy and operate effectively in a wide range of environments.
In addition to their role in real-time decision-making, RNNs are also used for predictive modeling in autonomous driving. By analyzing historical data and patterns in driving behavior, RNNs can anticipate potential hazards, plan optimal routes, and optimize driving strategies to improve efficiency and safety.
Overall, recurrent neural networks play a critical role in the development of autonomous driving technology, enabling vehicles to process complex sensory inputs, make intelligent decisions, and adapt to changing environments. As research and development in this field continue to advance, RNNs are expected to play an even greater role in shaping the future of autonomous transportation, making roads safer and more efficient for everyone.
#Role #Recurrent #Neural #Networks #Autonomous #Driving #Technology,rnn
Leave a Reply