Your cart is currently empty!
Transfer Learning for Multiagent Reinforcement Learning Systems [Synthesis Lectu
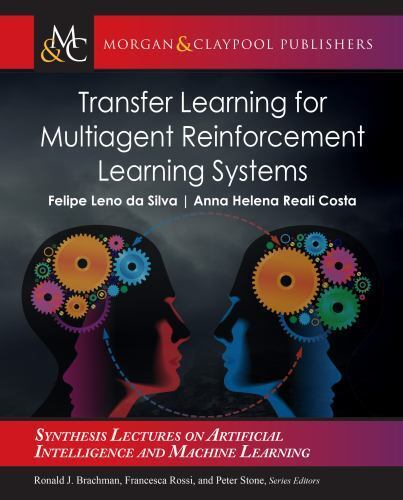
Transfer Learning for Multiagent Reinforcement Learning Systems [Synthesis Lectu
Price : 44.00
Ends on : N/A
View on eBay
re]
In the field of multiagent reinforcement learning (MARL), transfer learning has emerged as a promising approach to improve the performance of agents in complex and dynamic environments. Transfer learning involves transferring knowledge from a source task to a target task, allowing agents to leverage past experiences to accelerate learning in new environments.
In this post, we will explore the concept of transfer learning in the context of MARL systems. We will discuss the challenges and opportunities of applying transfer learning techniques to multiagent settings, and how it can enhance the scalability and generalization capabilities of MARL systems.
We will also delve into the different approaches and algorithms that have been developed to facilitate transfer learning in MARL systems, including model-based and model-free methods, as well as techniques such as policy transfer, value function transfer, and knowledge distillation.
Overall, transfer learning holds great potential for enhancing the performance and adaptability of multiagent systems in complex and dynamic environments. By leveraging past experiences and knowledge, agents can learn more efficiently and effectively, leading to improved coordination, collaboration, and overall performance in multiagent tasks.
Stay tuned for our upcoming post where we will delve deeper into the technical aspects of transfer learning in MARL systems and discuss the latest advancements in the field.
#Transfer #Learning #Multiagent #Reinforcement #Learning #Systems #Synthesis #Lectu
Leave a Reply