Your cart is currently empty!
Transfer Learning for Natural Language Processing
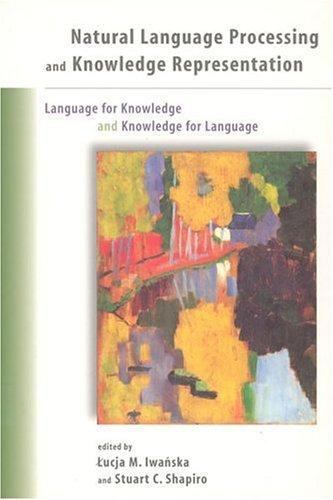
Transfer Learning for Natural Language Processing
Price : 9.45
Ends on : N/A
View on eBay
Transfer learning is a powerful technique in the field of Natural Language Processing (NLP) that allows us to leverage pre-trained models and adapt them to new tasks or datasets. By using transfer learning, we can save time and resources by reusing knowledge learned from large-scale datasets.
There are different approaches to transfer learning in NLP, such as fine-tuning pre-trained language models, feature extraction, and domain adaptation. Fine-tuning involves taking a pre-trained model, such as BERT or GPT-3, and training it on a smaller, domain-specific dataset to improve its performance on a specific task. Feature extraction, on the other hand, involves using the pre-trained model as a feature extractor and feeding the extracted features into a new model for a specific task.
Domain adaptation is another form of transfer learning that aims to adapt a pre-trained model to a new domain or dataset by fine-tuning it on a small amount of labeled data from the new domain.
Transfer learning has been widely used in various NLP tasks, such as sentiment analysis, named entity recognition, machine translation, and text classification. By leveraging pre-trained models and transfer learning techniques, researchers and developers can achieve state-of-the-art performance on NLP tasks with less data and computational resources.
In conclusion, transfer learning is a valuable tool in NLP that can help us achieve better performance on various tasks by leveraging pre-trained models and adapting them to new datasets. By understanding and applying transfer learning techniques, we can accelerate the development of NLP applications and improve the efficiency of model training.
#Transfer #Learning #Natural #Language #Processing
Leave a Reply