Your cart is currently empty!
Understanding Complex Datasets: Data Mining With Matrix Decompositions
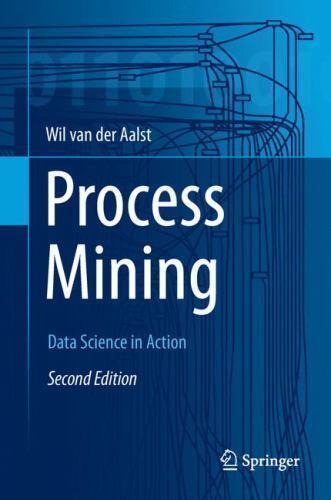
Understanding Complex Datasets: Data Mining With Matrix Decompositions
Price : 119.55
Ends on : N/A
View on eBay
Data mining is a powerful technique used to extract valuable insights and patterns from large and complex datasets. One of the key methods used in data mining is matrix decompositions. Matrix decompositions involve breaking down a matrix into simpler components to better understand the underlying structure of the data.
There are several types of matrix decompositions that can be used in data mining, such as Singular Value Decomposition (SVD), Principal Component Analysis (PCA), and Non-negative Matrix Factorization (NMF). Each of these methods has its own strengths and weaknesses, and can be used in different scenarios depending on the nature of the data.
SVD is a widely used matrix decomposition technique that can be used for dimensionality reduction, denoising, and collaborative filtering. PCA is another popular method that is used to reduce the dimensionality of a dataset while preserving as much variance as possible. NMF is often used in text mining and image processing to extract meaningful features from the data.
By understanding and applying matrix decompositions in data mining, researchers and analysts can uncover hidden patterns and relationships in their datasets that may not be apparent through traditional analysis techniques. This can lead to more accurate predictions, better decision-making, and valuable insights that can drive business success.
In conclusion, matrix decompositions are a valuable tool in the data mining toolkit that can help analysts make sense of complex datasets and extract valuable insights. By learning and applying these techniques effectively, researchers can unlock the full potential of their data and make more informed decisions.
#Understanding #Complex #Datasets #Data #Mining #Matrix #Decompositions
Leave a Reply