Your cart is currently empty!
Understanding the Key Principles of Machine Learning: A Triple Perspective
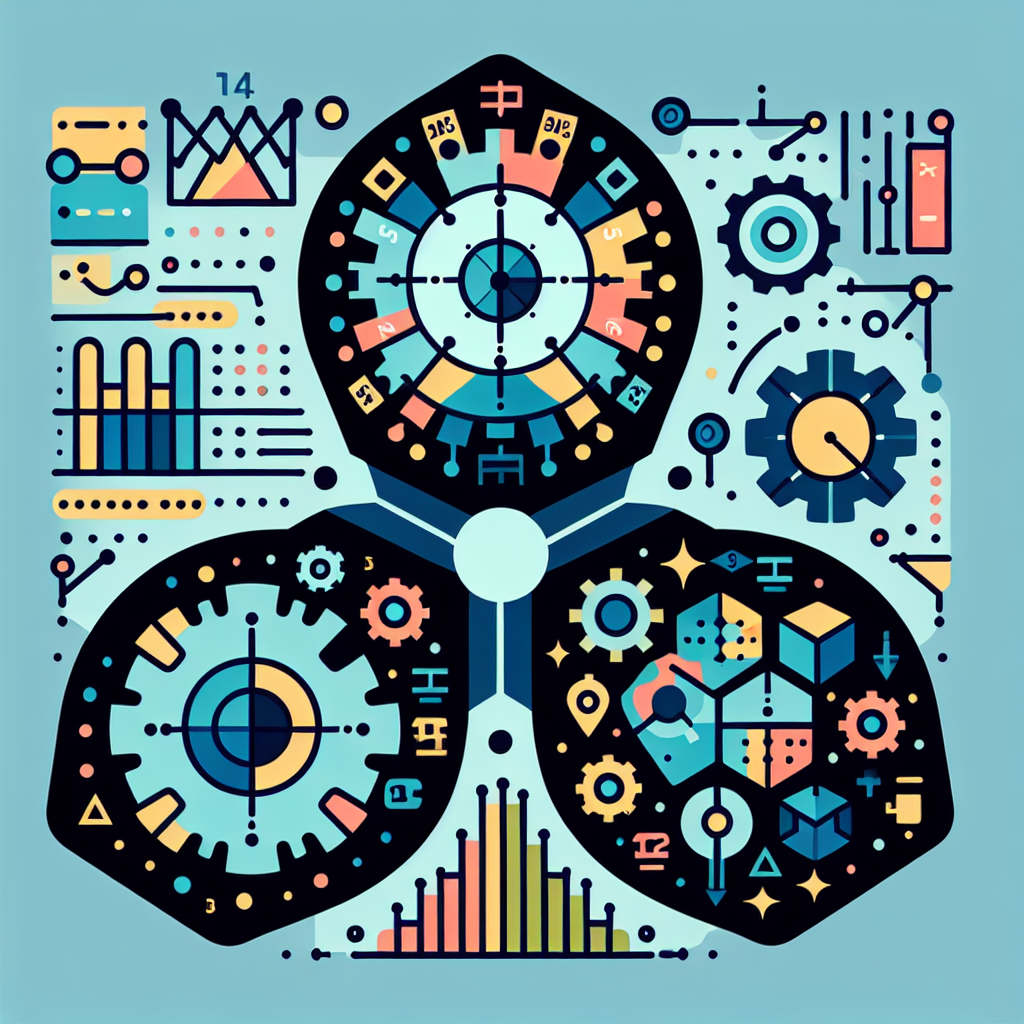
Machine learning is a rapidly growing field in technology that is revolutionizing the way we interact with computers and data. By using algorithms and statistical models, machine learning allows computers to learn from and make predictions or decisions based on data without being explicitly programmed to do so. In this article, we will explore the key principles of machine learning from three different perspectives: the technical perspective, the business perspective, and the ethical perspective.
From a technical perspective, the key principles of machine learning revolve around algorithms, data, and models. Algorithms are the mathematical formulas that enable computers to learn from data and make predictions or decisions. Data is the fuel that drives machine learning, as algorithms need large amounts of high-quality data to learn patterns and make accurate predictions. Models are the end result of the machine learning process, representing the learned patterns and relationships in the data that can be used for prediction or decision-making.
One of the key principles of machine learning is the concept of training and testing data. Training data is used to teach the machine learning algorithm to recognize patterns and make predictions, while testing data is used to evaluate the performance of the model on unseen data. This process is crucial for ensuring that the model is accurate and reliable in making predictions.
Another key principle of machine learning is the concept of overfitting and underfitting. Overfitting occurs when a model learns the noise in the training data rather than the underlying patterns, leading to poor performance on new data. Underfitting, on the other hand, occurs when a model is too simple to capture the complexities in the data, also leading to poor performance. Balancing between overfitting and underfitting is essential for building a reliable machine learning model.
From a business perspective, the key principles of machine learning center around value creation and competitive advantage. Machine learning can help businesses make better decisions, improve processes, and create new products and services based on data-driven insights. By leveraging machine learning, businesses can optimize operations, personalize customer experiences, and drive innovation.
One of the key principles of machine learning in business is the importance of understanding the problem domain and defining clear objectives. Without a clear understanding of the problem to be solved and the desired outcomes, machine learning projects can easily go off track or fail to deliver value. It is crucial for businesses to align machine learning initiatives with their strategic goals and priorities to ensure success.
Another key principle of machine learning in business is the need for cross-functional collaboration and communication. Machine learning projects often require a multidisciplinary team of data scientists, engineers, domain experts, and business stakeholders to work together towards a common goal. Effective communication and collaboration are essential for ensuring that machine learning projects are successful and deliver value to the business.
From an ethical perspective, the key principles of machine learning revolve around fairness, accountability, and transparency. Machine learning algorithms have the potential to perpetuate bias, discrimination, and inequality if not carefully designed and implemented. It is important for developers, researchers, and policymakers to consider the ethical implications of machine learning and take steps to mitigate potential harms.
One of the key principles of machine learning ethics is the concept of fairness and bias. Machine learning algorithms can inadvertently learn biases present in the data, leading to discriminatory outcomes or unfair treatment of certain groups. It is crucial for developers to proactively identify and address biases in the data and algorithms to ensure fair and equitable outcomes for all users.
Another key principle of machine learning ethics is the concept of accountability and transparency. Developers and organizations should be transparent about how machine learning algorithms are used, what data is being collected and processed, and how decisions are made. Accountability mechanisms should be put in place to ensure that individuals can understand and challenge the outcomes of machine learning algorithms.
In conclusion, understanding the key principles of machine learning from a triple perspective – technical, business, and ethical – is essential for building reliable, valuable, and ethical machine learning systems. By considering these perspectives and principles, developers, businesses, and policymakers can harness the power of machine learning to drive innovation, create value, and promote ethical use of technology.
#Understanding #Key #Principles #Machine #Learning #Triple #Perspective,principles of machine learning: the three perspectives
Leave a Reply