Your cart is currently empty!
Unleashing the Potential of Recurrent Neural Networks in Image Recognition
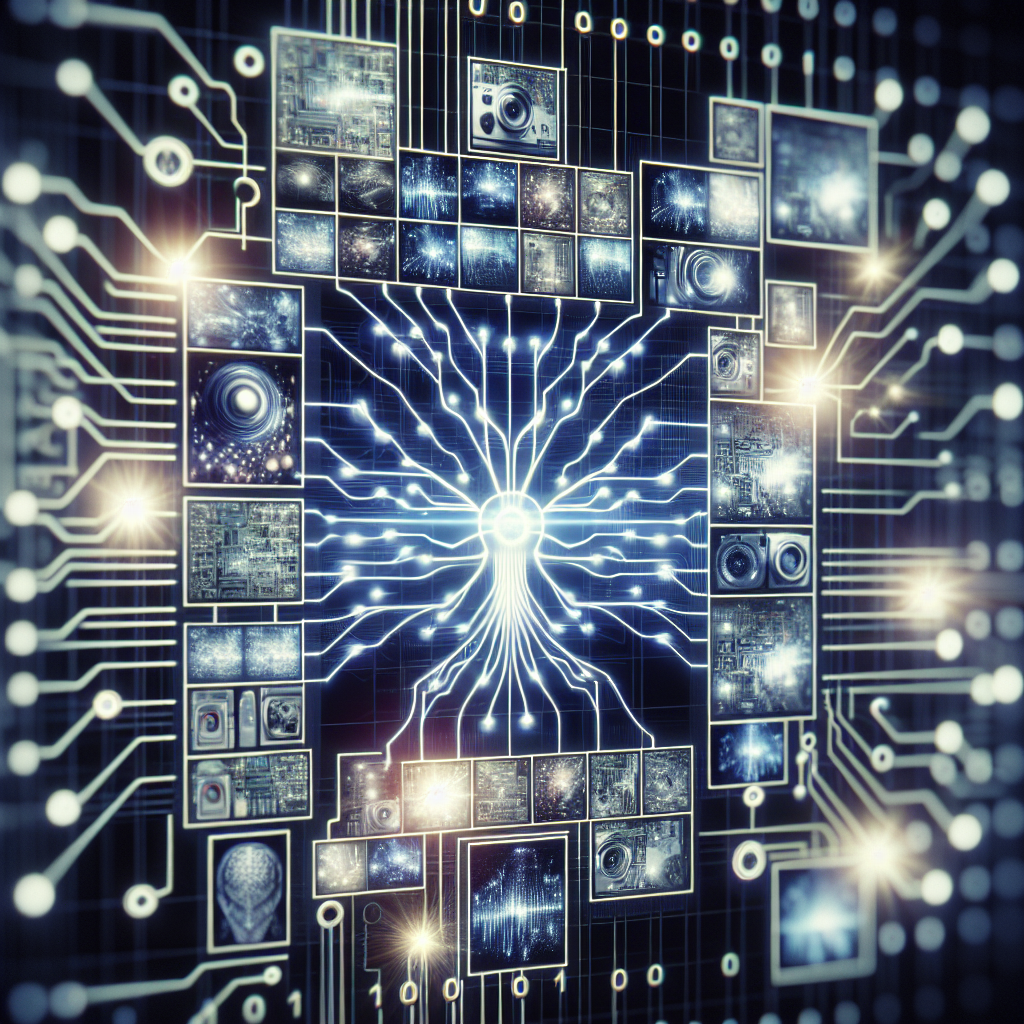
Image recognition is a rapidly growing field in artificial intelligence, with applications ranging from facial recognition to autonomous driving. One of the key technologies driving this progress is recurrent neural networks (RNNs), which have shown great promise in recognizing and classifying images.
RNNs are a type of neural network that is designed to handle sequential data, making them particularly well-suited for tasks like image recognition where the order of information is important. Traditional neural networks, such as convolutional neural networks (CNNs), are excellent at recognizing patterns in images, but they struggle to capture the temporal dependencies that are present in many real-world scenarios.
By contrast, RNNs are able to remember information from previous time steps and use it to make predictions about the current input. This makes them especially useful for tasks like video analysis, where the context of a scene can change rapidly over time.
One of the key advantages of RNNs in image recognition is their ability to handle variable-length inputs. This is particularly important in tasks like object detection, where the number and size of objects in an image can vary greatly. RNNs can adapt to these variations by dynamically adjusting the size of their internal memory, allowing them to process images of any size or shape.
Another important feature of RNNs is their ability to learn long-range dependencies in data. This is crucial for tasks like image captioning, where the relationship between different objects in a scene may be spread out across several time steps. RNNs can capture these complex relationships by learning to associate different parts of an image with each other, allowing them to generate accurate and meaningful captions.
Despite their potential, RNNs still face several challenges in image recognition. One of the main issues is the vanishing gradient problem, where the gradients of the network become very small and prevent the model from learning effectively. This can be mitigated by using techniques like gradient clipping and long short-term memory (LSTM) cells, which are designed to better capture long-term dependencies in data.
Overall, RNNs have shown great promise in image recognition and have the potential to revolutionize the field. By leveraging their ability to handle sequential data and learn long-range dependencies, researchers can push the boundaries of what is possible in image recognition. As these technologies continue to develop, we can expect to see even more impressive applications of RNNs in fields like healthcare, security, and entertainment.
#Unleashing #Potential #Recurrent #Neural #Networks #Image #Recognition,rnn
Leave a Reply