Your cart is currently empty!
Unlocking the Potential of GNN: How Graph Neural Networks Are Transforming Data Analysis
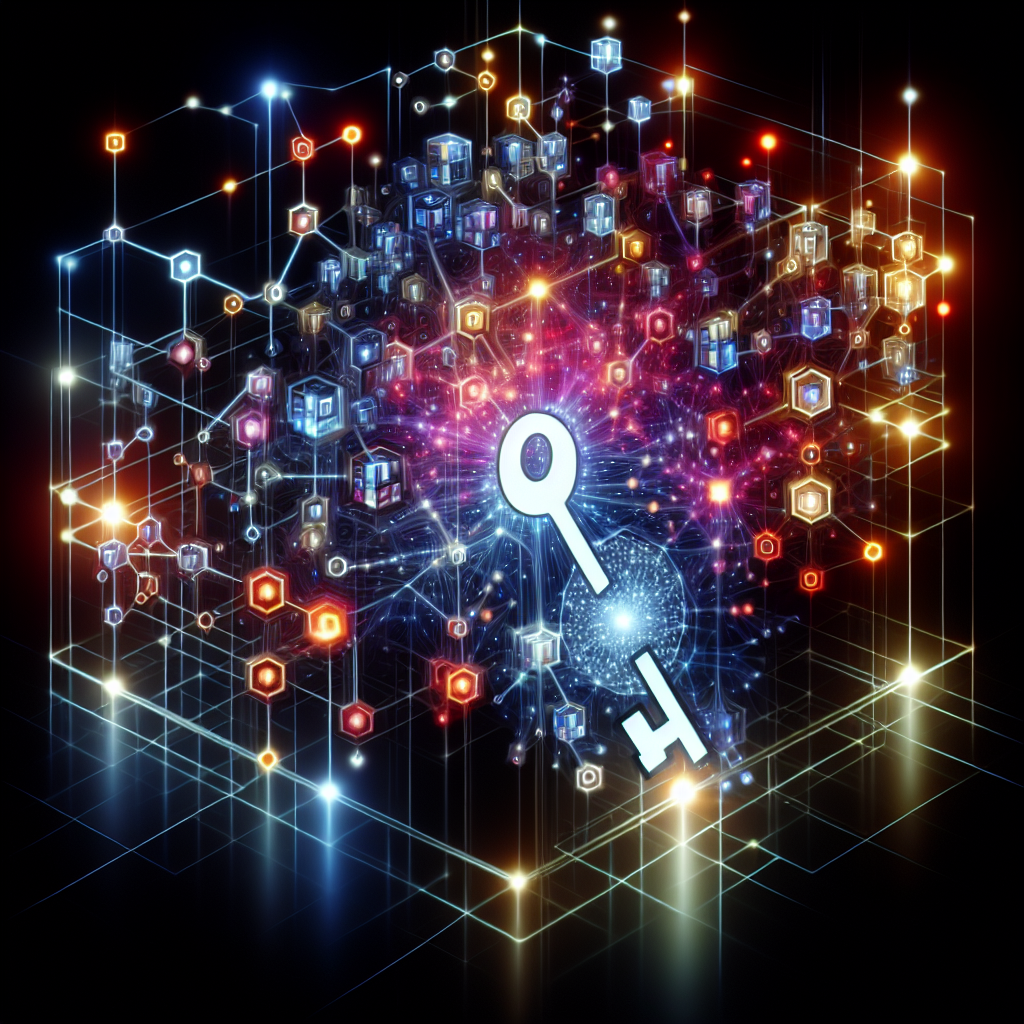
Graph Neural Networks (GNNs) have emerged as a powerful tool for analyzing and processing data in a variety of fields, including social networks, recommendation systems, and bioinformatics. By representing data as graphs, GNNs are able to capture complex relationships and dependencies between different entities, allowing for more accurate and efficient data analysis.
One of the key advantages of GNNs is their ability to learn from both the structure of the graph and the features associated with each node. This means that GNNs can take into account not only the connections between nodes, but also the attributes of each node, such as its location, age, or preferences. This allows GNNs to make more informed decisions and predictions based on the data available.
In the field of social networks, GNNs have been used to analyze and predict the behavior of users, detect communities and clusters within the network, and recommend connections between users. By leveraging the graph structure of social networks, GNNs are able to uncover hidden patterns and insights that traditional machine learning algorithms may overlook.
In recommendation systems, GNNs have proven to be highly effective at identifying relevant items for users based on their preferences and browsing history. By modeling the relationships between users and items as a graph, GNNs are able to make personalized recommendations that take into account not only the user’s past interactions, but also the connections between similar users and items.
In bioinformatics, GNNs have been used to analyze biological networks, such as protein-protein interaction networks and gene regulatory networks. By modeling these networks as graphs, GNNs are able to identify important nodes and edges, predict protein functions, and uncover potential drug targets for diseases.
Overall, GNNs have the potential to revolutionize data analysis by unlocking the power of graph data. By leveraging the rich structure and relationships inherent in graphs, GNNs are able to extract valuable insights and make more accurate predictions than traditional machine learning algorithms. As the field of GNNs continues to evolve, we can expect to see even more innovative applications and advancements in data analysis.
#Unlocking #Potential #GNN #Graph #Neural #Networks #Transforming #Data #Analysis,gnn
Leave a Reply