Your cart is currently empty!
Unsupervised Learning: Foundations of Neural Computation
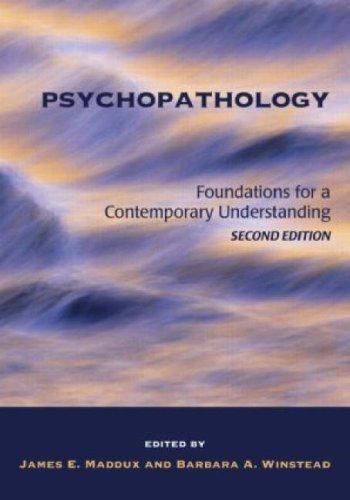
Unsupervised Learning: Foundations of Neural Computation
Price : 47.07
Ends on : N/A
View on eBay
Unsupervised Learning: Foundations of Neural Computation
Unsupervised learning is a crucial component of artificial intelligence and machine learning, providing a way for algorithms to learn patterns and relationships in data without the need for labelled examples. This type of learning is essential for tasks such as clustering, dimensionality reduction, and anomaly detection.
In the realm of neural computation, unsupervised learning plays a key role in mimicking the way the human brain processes information. By allowing neural networks to learn from raw data without explicit guidance, unsupervised learning enables them to discover hidden structures and features that may not be apparent to the naked eye.
One popular technique in unsupervised learning is the autoencoder, which is a neural network that is trained to reconstruct its input data. By compressing the input data into a lower-dimensional representation and then decoding it back to its original form, autoencoders can learn meaningful representations of the input data without any explicit supervision.
Another common approach in unsupervised learning is clustering, which involves grouping similar data points together based on their features. Clustering algorithms such as K-means and hierarchical clustering can be used to identify patterns and relationships in data that can be used for tasks such as customer segmentation or anomaly detection.
Overall, unsupervised learning is a powerful tool in the field of neural computation, providing a way for algorithms to learn from raw data and discover hidden structures and patterns. By understanding the foundations of unsupervised learning, researchers and practitioners can leverage these techniques to build more intelligent and efficient systems.
#Unsupervised #Learning #Foundations #Neural #Computation
Leave a Reply