Your cart is currently empty!
Utilizing Gan to Improve NLP Models for PDF Text Extraction
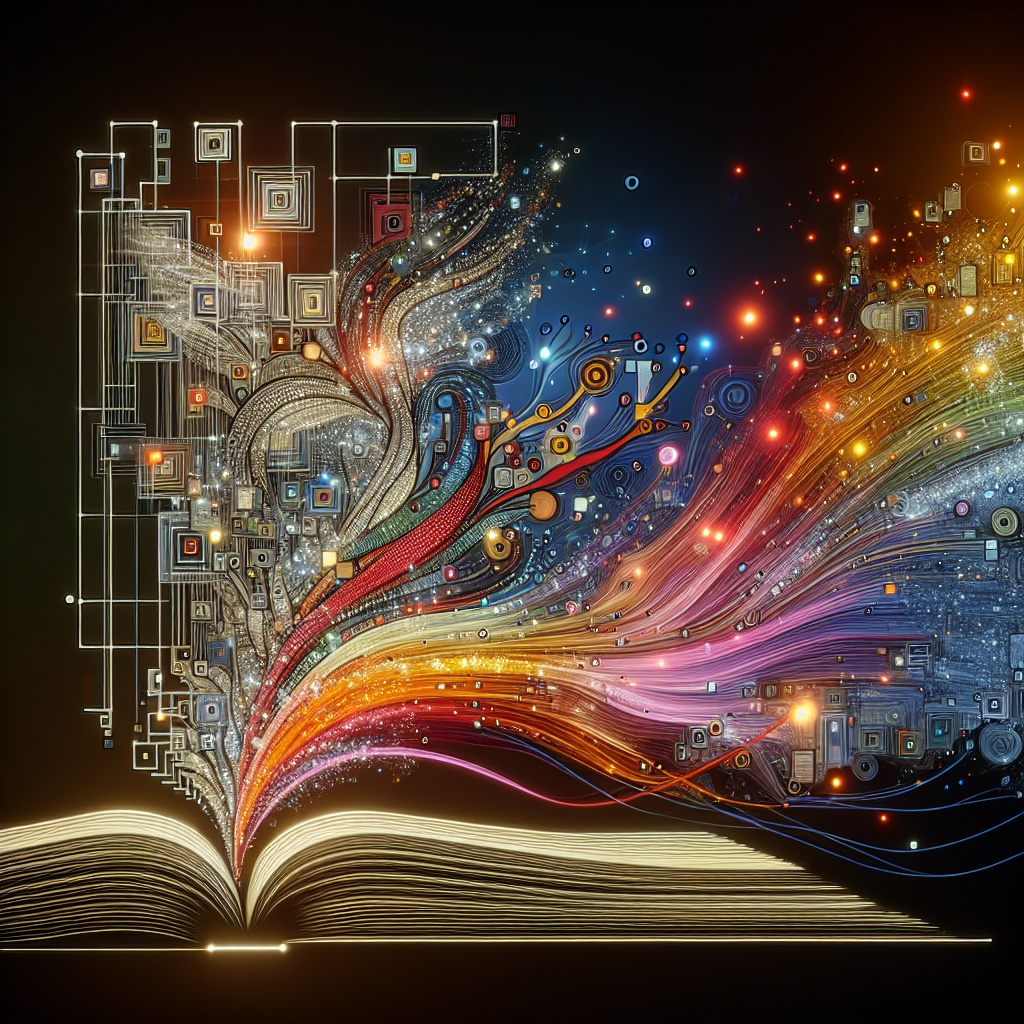
Natural Language Processing (NLP) is a branch of artificial intelligence that focuses on the interaction between computers and human language. One common application of NLP is text extraction from PDF documents, which involves converting the text from a PDF file into a machine-readable format. This process is crucial for tasks such as information retrieval, text analysis, and data mining.
Recently, researchers have been exploring the use of Generative Adversarial Networks (GANs) to improve NLP models for PDF text extraction. GANs are a type of neural network that consists of two networks – a generator and a discriminator – which work together to generate realistic data. GANs have been successfully used in various applications, such as image generation and style transfer, and now they are being applied to NLP tasks as well.
One of the main advantages of using GANs for PDF text extraction is their ability to generate synthetic text data that closely resembles real text. This can be particularly useful when dealing with PDF documents that have poor quality scans or low-resolution images, as GANs can help fill in missing or distorted text. Additionally, GANs can be trained on a large corpus of text data to improve the performance of NLP models, leading to more accurate and reliable text extraction results.
Another benefit of using GANs for PDF text extraction is their ability to learn and adapt to different types of document layouts and formats. Traditional NLP models may struggle with extracting text from PDFs that have complex structures, such as multi-column layouts or tables. By training GANs on a diverse set of PDF documents, researchers can improve the robustness and flexibility of NLP models, making them more versatile in handling various types of documents.
Furthermore, GANs can be used to enhance the pre-processing and data augmentation steps in NLP pipelines for PDF text extraction. By generating synthetic text data, GANs can help improve the quality and quantity of training data, leading to better model performance and generalization. This can be particularly beneficial for tasks that require a large amount of annotated text data, such as named entity recognition or sentiment analysis.
In conclusion, utilizing GANs to improve NLP models for PDF text extraction has the potential to revolutionize the way we extract and analyze text data from documents. By leveraging the power of GANs to generate synthetic text data, researchers can enhance the performance, robustness, and versatility of NLP models, leading to more accurate and efficient text extraction results. As the field of NLP continues to evolve, we can expect to see more innovative applications of GANs in text extraction tasks, paving the way for new advancements in artificial intelligence and document processing.
#Utilizing #Gan #Improve #NLP #Models #PDF #Text #Extraction,gan)
to natural language processing (nlp) pdf
Leave a Reply